3D facial imaging aids early autism detection
Autism is a spectrum of closely related disorders diagnosed in patients who exhibit a shared core of symptoms that include delays in learning, communication and social interaction. Early detection of autism in children is the key for treatments to be most effective and produce the best outcomes.
Today, a number of diagnostic tests are used to evaluate these patients including the Autism Diagnostic Interview-Revised (ADI-R; http://bit.ly/1KNJyKw) and the Autism Diagnostic Observation Schedule (ADOS; http://bit.ly/1ynMerT) which measure impairment in social functioning, communication and repetitive behavior. By interviewing and observing patients using standardized methods such as these, a diagnosis of possible autism can be made.
However, rather than perform such extensive interviews and observations, recent work by Dr. Ye Duan, an Associate Professor of Computer Science at the University of Missouri (MU; Columbia, MO, USA; www.engineering.missouri.edu) has shown that, using 3D facial imaging measurement and statistical analysis techniques may lead to a screening tool for young children and provide clues to its genetic causes.
"Detecting the specific facial traits of the face of a child with autism," says Duan "may help define the facial structures common to children with autism and potentially enable early screening for the disorder."
Duan, working with Judith Miles, Professor Emerita of child health-genetics in the MU Thompson Center for Autism and Neurodevelopmental Disorders, used a 3dMDcranial system from 3dMD (Atlanta, GA, USA; www.3dmd.com) to capture 3D facial images of 8-12 year-old children. While one group of children had been diagnosed with autism by the Thompson Center; the other group consisted of typically developing children.
After 3D facial images were captured by the 3dMDcranial system, 3dMD software was used to obtain 3D coordinate data for a set of 19 facial landmarks. Geodesic (surface) facial distances between all possible pairs of the nineteen landmarks were then measured, resulting in a total of 171 facial distance features.
To identify autistic children with similar morphological facial features, the finite unlabeled data set of features was applied to four different clustering algorithms: k-means, self-organizing feature maps (SOM), expectation maximization (EM) and partitioning around medoids (PAM). The performance of these clustering algorithms was then evaluated using the cluster validity analysis platform (CVAP) program found in MATLAB software from MathWorks (Natick, MA, USA; www.mathworks.com). Based on this analysis, the k-means clustering algorithm was chosen as the optimal clustering algorithm.
To classify the data from this clustering algorithm, three classification models that consisted of support vector machines (SVMs), a neural network layer perceptron (MLP) model and a random forest (RF) network were applied. However, because using 171 facial distance features increases the likelihood of feature redundancy, it was necessary to remove any irrelevant or redundant features.
To determine which features were significant and discriminant, a parallel scatter search algorithm, a best first search algorithm and a linear forward selection feature selection algorithm were all applied to the data. A minimum set of 31 features were then derived and re-applied to the classification models to confirm the significance of these features. This resulted in improved classification performance for both the SVM and RF models and equal performance for the MLP.
To determine whether the results of the cluster analysis can be used as an indicator of autism, ADI-R and ADOS scores for autistic and normally developing children patients were compared with the results from the three clusters generated by the classification models. The resulting correlation between autism and facial measurement classification then affirmed that the technique can be used for autism diagnosis.
About the Author
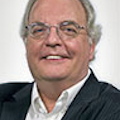
Andy Wilson
Founding Editor
Founding editor of Vision Systems Design. Industry authority and author of thousands of technical articles on image processing, machine vision, and computer science.
B.Sc., Warwick University
Tel: 603-891-9115
Fax: 603-891-9297