Drone-based SWIR camera inspects solar panels in daylight
Defects and faults in photovoltaic (PV) solar panels lead to production loss or inoperability, making swift identification of the issue imperative. Cell cracks, shunts, and broken cell interconnections cannot be seen with the naked eye, but drones equipped with cameras offer an effective method for daytime detection of defects that negatively impact solar panels.
Electroluminescence (EL) imaging produces highly detailed PV diagnosis data and is deployed often in PV solar panel inspection applications. EL offers more accurate results than infrared thermography in fault identification because the images provide resolution in the semiconductor material level. The technique is commonly practiced indoors or outdoors from dusk to dawn, because crystalline silicon luminescence has a signal several orders of magnitude lower than sunlight.
Related: Novel sensor process enables SWIR and visible light capture on single chip
Image acquisition
Seeking a method to effectively capture images in daylight, a team of researchers from the Technical University of Denmark (DTU) lead by Gisele Alves dos Reis Benatto and Peter Behrensdorff Poulsen developed a drone-based image capture system (Figure 1). In bright sunlight conditions, using daylight filters and subtracting (i.e. calculating the difference of each pixel) a single background image to obtain a clear EL image is usually not sufficient. With the EL signal intensity so much lower than sunlight, this leads to a very low signal-to-noise ratio.
To compensate for this, the team acquired many EL and background image pairs taken at the same fixed position while the PV panels where electrically modulated and added postprocessing steps to produce images consisting of the average and subtraction of the background image for each pair. Doing so increased the signal-to-noise ratio to an acceptable level, according to the researchers.
For successful daylight EL imaging, images must be acquired fast and with short exposure times to avoid motion blur and saturation due to sunlight. InGaAs-based detectors meet these requirements, so the team chose an Owl 640 640 x 512 shortwave infrared (SWIR) camera from Raptor Photonics (Larne, Co Antrim, UK; www.raptorphotonics.com). The camera paired with a 25 mm fixed focus sapphire lens and an 1150 nm bandpass filter with 50 nm full width at half maximum (FWHM) to avoid detecting light from the sun and surroundings.
In addition to the camera, the EL/background image pair acquisition apparatus comprises a hexacopter drone, and an Apalis TK1 NVIDIA Tegra K1 SoC-based embedded PC from Toradex (Seattle, WA, USA; www.toradex.com) for camera control, live image feedback, and image storage. The drone connects to computer on the ground via two Wi-Fi channels for better signal stability, while the drone controller software allows semiautonomous flights according to regulations on safety.
Navigating approximately four meters from the panels, the camera acquired images of a mechanically-stressed PV module with 36 multicrystalline 15.6 x 15.6 cm cells arranged in a 6 x 6 cell matrix at a rate of 120 fps in the 1125 to 1175 nm range in testing in Denmark (Figure 2).
Electrical modulation and postprocessing
All images store locally in the embedded PC and are used for postprocessing after image acquisition. Even with appropriate hardware, further challenges exist for enhancing the signal-to-noise of images taken from the drone, particularly those acquired while in motion.
After image acquisition flights, images transfer from the embedded PC to a desktop PC for postprocessing using algorithms developed in MATLAB from MathWorks (Natick, MA, USA; www.mathworks.com). Image processing steps for stationary daylight EL include EL/background image separation, averaging, background subtraction, perspective correction, and signal-to-noise average calculation. For EL/background images acquired during movement—as this the case with drone acquisition—additional steps must be performed, including PV panel edge detection and segmentation in each image of the sequence, as well as motion compensation of the whole image sequence.
These steps take place before EL/background image separation and require highly accurate and robust image processing algorithms. In each frame, algorithms detect the panel and segment it from the rest of the image content, allowing for compensation for the motion between different frames and registering across all images of the sequence with a full search block matching algorithm. By performing averaging and background subtraction, the images are denoised.
Related: Unique image processing algorithms increase drone reaction speeds
The number of images required to improve signal-to-noise ratio until the recommended quality depends on factors such as sun irradiance and EL/background image, but spatial match represents the most important factor for image improvement, according to the researchers. When spatial match does not present a problem, such as in the case of stationary daylight EL, 16 image pairs prove sufficient for visually acceptable EL images for cell crack diagnosis, for example. Image sequences acquired under high irradiances tend to provide smaller EL/background image deltas, but approximately 50 well spatially matched image pairs overcome this drawback and provide good quality images for cell crack detection.
The team applied modulated electrical waveforms to the images using DC square waveform and AC+DC DC sinusoidal methods. For DC electrical modulation, a bi-directional SM 1500-CP30 DC power supply from Delta Elektronika (Zierikzee, Netherlands; www.delta-elektronika.nl/en) generated a modulated voltage bias for the PV panels while an AC6804A AC power source from Keysight Technologies (Santa Rosa, CA, USA; www.keysight.com) was used for modulated bias to avoid strong sun irradiance interferences in the bias signal.
AC+DC electrical modulation offered a more reproducible mean pixel value delta between EL and background images than DC electrical modulation, and DC modulation presented higher delta magnitude than AC+DC modulation (Figure 3). When imaging outdoors while in motion, the images were of lower quality compared with outdoor stationary because of a mismatch in positioning the image pairs acquired in motion. A positioning mismatch in subpixel level prevented optimal quality improvement in terms of signal-to-noise ratio and represented the main issue for low quality images in the drone-based EL imaging, according to the team.
Test results show that the algorithms for improving image quality need further programming steps for a drone-based EL acquisition system to perform utility inspections at scale, but the overall project shows promise for drone-based inspection of PV panels in daylight using SWIR cameras.
About the Author
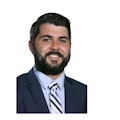
James Carroll
Former VSD Editor James Carroll joined the team 2013. Carroll covered machine vision and imaging from numerous angles, including application stories, industry news, market updates, and new products. In addition to writing and editing articles, Carroll managed the Innovators Awards program and webcasts.