Deep-learning robot teaches itself how to grasp objects
Researchers fromCarnegie Mellon University have equipped an industrial robot with deep-learning capabilities, which has enabled it to grasp objects from a table, learning from trial and error.
Lerrel Pinto and Abhinav Gupta from Carnegie Mellon chose a Baxter industrial robot for their research. Baxter is a two-armedforce-limited robot from Rethink Robotics. Baxter is a dual-armed collaborative robot with three cameras and a Microsoft Kinect infrared sensor that is employed to detect whether a human operator is within a 1.5 – 15 in. range of a machine, as the robot is generally intended to be used in automation applications. Each arm of the robot has a standard gripper and a camera to allow the robot to see what it is grasping.
Pinto and Gupta, according toMIT, programmed Baxter to grasp an object by isolating it from nearby objects, then to pick a random point in that area, rotate the grippers to a certain angle, and then move the grippers down vertically to attempt a grasp. The robot then lifts its arm and determines whether the grasp was successfully by using its force sensors. For each point, Baxter repeats the grasping process 188 times, each time after rotating the gripping angle by 10°.
In order for the robot to teach itself how to grasp, Pinto and Gupta placed a variety of objects on the table in front of it and let it run for up to 10 hours a day, without any intervention. Its deep-learning approach was based on a neural network that was pretrained in object recognition, which gave Baxter some essential skills to start with. Two of the layers of the network were devoted to learning to grasp in these random experiments, however.
Additionally, Baxter was equipped with a second stage oflearning to improve its skills. With the robot’s new set of skills, the team tested Baxter on a new set of objects, some of which Baxter had already seen and others that were newly-introduced. During more than 700 hours of testing, Baxter performed nearly 50,000 grasps on 150 different objects, each time learning whether the approach was successful or not. Items included a TV remote control, plastic toys, and other small objects. This produced a significant body of learning, which allowed the robot to predict whether or not a grasp would be successful almost 80% of the time.
Baxter’s approach, compared to other approaches such as a common sense approach in which the robot was programmed to pick up an object near its center, at its narrowest point while avoiding areas that were too narrow to grip, proved to be more successful. (80% compared to 62% of the aforementioned approach.)
Next steps, in terms of improving the robot’s ability to grasp, will include learning how hard to grip, according to the MIT article, which suggests that the ultimate test for Baxter may be the toothpaste challenge—the successful placement of a pea-sized blob of toothpaste on a toothbrush.
View a Carnegie Mellonacademic paper.
View the MIT article.
Share your vision-related news by contactingJames Carroll, Senior Web Editor, Vision Systems Design
To receive news like this in your inbox,click here
Join ourLinkedIn group | Like us on Facebook | Follow us on Twitter| Check us out on Google +
About the Author
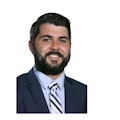
James Carroll
Former VSD Editor James Carroll joined the team 2013. Carroll covered machine vision and imaging from numerous angles, including application stories, industry news, market updates, and new products. In addition to writing and editing articles, Carroll managed the Innovators Awards program and webcasts.