The technical landscape for processors and sensors for embedded computer vision applications has changed tremendously over the past five years and will continue to change dramatically over the next five years.
There’s been an incredible acceleration in innovation in these spaces, driven by rapidly growing markets. For example, Tractica forecasts a 25% annual increase in revenue for computer vision hardware, software, and services between now and 2025, reaching $26 billion.
Arguably, the most important ingredient driving the widespread deployment of visual perception is better processors. Vision algorithms typically have huge appetites for computing performance. Achieving the required levels of performance with acceptable cost and power consumption is a common challenge, particularly as vision is deployed into cost-sensitive and battery-powered devices.
Fortunately, in the past few years there’s been an explosion in the development of processors tuned for computer vision applications. These purpose-built processors are now coming to market, delivering huge improvements in performance, cost, energy efficiency and ease of development.
Progress in efficient processors has been boosted by the growing adoption of deep learning for two reasons. First, deep learning algorithms tend to require even more processing performance than conventional computer vision algorithms. Second, the most widely used deep learning algorithms share many common characteristics, which simplifies the task of designing a specialized processor intended to execute these algorithms efficiently. In contrast, conventional computer vision algorithms exhibit extreme diversity.
Today, typically, computer vision applications use a combination of a general-purpose CPU and a specialized parallel co-processor. Historically, GPUs have been the most popular type of co-processor because they were widely available and supported with good programming tools.
These days, there’s a much wider range of co-processor options, with newer types of co-processors typically offering significantly better efficiency compared to GPUs. The trade-off is that these newer processors are less widely available, less familiar to developers and not yet as well supported by mature development tools.
According to the most recent Embedded Vision Alliance developer survey, completed in November 2018, nearly one-third of developers creating vision-related products are using deep learning-specific co-processors. This is remarkable, considering that deep learning-specific processors didn't exist a few years ago.
Sensors are also evolving very rapidly. The 2D image sensors found in many vision systems enable a tremendous breadth of vision capabilities. But adding depth information can be extremely valuable. For example, the ability to discern not only lateral motion but also motion perpendicular to the sensor greatly expands the variety of gestures that a system can recognize.
In other applications, depth information enhances accuracy. In face recognition, for example, depth sensing is valuable in determining that the object being sensed is an actual face, versus a photograph. And the value of depth information is obvious in moving systems, such as mobile robots and automobiles.
Historically, depth sensing has been an exotic, expensive technology, but this has changed dramatically in the past few years. The use of optical depth sensors in the Microsoft Kinect, and more recently in mobile phones, has catalyzed a rapid acceleration in innovation, resulting in depth sensors that are tiny, inexpensive and energy-efficient.
This change has not been lost on system developers. Thirty-four percent of developers participating in the Alliance’s most recent survey are already using depth perception, with another 29% (up from 21% a year ago) planning to incorporate depth in upcoming projects across a broad range of industries.
Our survey showed that there’s unprecedented growth in investment, innovation and deployment of practical computer vision technology across a broad range of markets. Because this market is relatively young, there’s always something new, and we expect to see many new processors and sensors at ourEmbedded Vision Summit next month in Santa Clara, CA. A white paper summarizing the survey results may be downloaded here, and I also gave an overview of the results in a recent presentation available here in video form and here as a slide deck PDF.
Jeff Bier
Founder, Embedded Vision Alliance
President, BDTI
About the Author
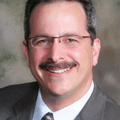
Jeff Bier
Founder, Embedded Vision Alliance
Jeff Bier is the founder of the Embedded Vision Alliance, a partnership of 90+ technology companies that works to enable the widespread use of practical computer vision. The Alliance’s annual conference, the Embedded Vision Summit (May 20-23, 2019 in Santa Clara, California) is the preeminent event where engineers, product designers, and business people gather to make vision-based products a reality.
When not running the Alliance, Jeff is the president of BDTI, an engineering services firm: for over 25 years BDTI has helped hundreds of companies select the right technologies and develop optimized, custom algorithms and software for demanding applications in audio, video, machine learning and computer vision. If you are choosing between processor options for your next design, need a custom algorithm to solve a unique visual perception problem, or need to fit demanding algorithms into a small cost/size/power envelope, BDTI can help.