Machine learning method automates subjective image quality tuning
Algolux, a Canadian company specializing in machine learning optimization platforms for autonomous vision, has announced the release of NaturalIQ, an artificial intelligence approach that enables users to automate subjective camera tuning by learning image quality preferences from their own natural image datasets.
Through its CRISP-ML software platform, Algolux—a winner of Gold and Silver level 2018 Innovators Awards—developed an automated approach to optimal image quality tuning by applying novel machine learning solvers and key performance indicators (KPI). This approach, according to the company, has shown to significantly reduce tuning time and cost, while improving scalability. However, Algolux notes, embedded camera providers still struggled to emulate best-in-class consumer cameras, resulting in many tuning iterations in the attempt to converge on the conflicting stakeholder objectives, and requires hard-to-find expertise.
NaturalIQ enables camera teams to tune against a dataset of their preferred natural images that represents the image quality outcome they desire, according to the Algolux press release. This image dataset can be created with photo editing tools or captured with a camera of choice, simplifying the subjective tuning process Once a meaningful image dataset is collected, the images are displayed and captured by the camera configuration being tuned, and NaturalIQ iteratively analyzes the camera output to determine how close the camera’s tuning parameter settings are to the goal defined by the image dataset and searches the parameter space to find the best settings. (Pictured above).
For camera teams with deep image quality tuning expertise, this reduces the effort and uncertainty involved with subjective image quality requirements. For teams with limited or even no tuning resources or expertise, NaturalIQ provides a more intuitive method differentiate their cameras and quickly respond to changing subjective requirements, according to Algolux.
Through its CRISP-ML software platform, Algolux developed an automated approach to optimal image quality tuning by applying novel machine learning solvers and key performance indicators (KPI). This approach, according to the company, has shown to significantly reduce tuning time and cost, while improving scalability. Coinciding with the release of NaturalIQ was the introduction of the latest version of CRISP-ML. This version, which aims to “significantly improve the effectiveness of camera teams to reach their objective image quality goals,” features a number of enhancements. These include an improved tuning methodology for increased usability and flexibility to achieve customer image quality goals, enhanced image quality tuning and optimization analysis from system to component level, and the accelerated bring-up of new and archived tuning projects.
“Algolux was the first to successfully automate the lengthy manual process of tuning ISP-based cameras by optimizing against objective image quality metrics, critical for many applications and further emphasized by industry initiatives, such as the IEEE P2020 Standard for Automotive System Image Quality,” said Robert Stead of Sense Media Group, Chair of the AutoSens Conference and IEEE P2020 Working Group. “NaturalIQ augments the objective CRISP-ML approach by learning from the customer’s set of ideal images to more predictably achieve a subjectively optimal camera tuning in a matter of hours or days.”
NaturalIQ is currently in initial trials with availability targeted for end 2018. Those interested in participating are encouraged to contact Algolux.
View more information on Algolux.
Share your vision-related news by contacting James Carroll, Senior Web Editor, Vision Systems Design
To receive news like this in your inbox, click here.
Join our LinkedIn group | Like us on Facebook | Follow us on Twitter
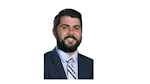
James Carroll
Former VSD Editor James Carroll joined the team 2013. Carroll covered machine vision and imaging from numerous angles, including application stories, industry news, market updates, and new products. In addition to writing and editing articles, Carroll managed the Innovators Awards program and webcasts.