AI and Rugged Edge Computing Are Fueling a Range of Applications
Edge computing has swiftly transitioned from an emerging technology to a critical component in modern industrial operations. The shift towards processing data at the edge of the network is a revolution, reducing reliance on centralized data centers and enabling real-time decision-making and data analysis closer to the data source. These abilities are driving adoption of artificial intelligence (AI) and machine learning (ML), technologies poised to redefine the current state of edge computing, its applications, and its future.
Rugged Edge Computing Paired with AI and ML
Edge computing involves deploying computing resources at the periphery of a network, close to where data is generated. Rugged edge computers push edge computing further. These systems are designed to operate reliably in the harshest industrial environments, where extreme temperatures, dust, debris, and vibrations are commonplace. From external enclosure to internal components, every element of a rugged edge computer is purpose-built through a combination of mechanical and thermal engineering to address environmental issues such as strong vibration, severe temperatures, and the presence of moisture or dirt.
Unlike traditional computing equipment, rugged edge computers provide continuous, reliable operation, ensuring consistent performance and minimizing downtime. This reliability is crucial in critical industrial applications and, when paired with artificial intelligence (AI) and machine learning (ML), is accelerating industry transformation. These technologies enhance real-time decision-making and operational efficiency, paving the way for smart factories and advanced automation applications, including those involving machine vision and imaging. This integration fosters deeper engineering engagement with industrial automation, igniting excitement around future possibilities. The system functions as an inference computer, tapping into hardware acceleration to handle data-heavy AI algorithms in real time—in scenarios away from the cloud and closer to data generation where IoT sensors reside.
Related: Rugged Computing Takes on the Battlefield Networking Challenge
Deploying Autonomous Delivery Trucks
The complex logistics sector offers an example. Committed to implementing automation, one trucking firm put its resources into developing self-driving delivery trucks. This shift altered its critical middle-mile phase, where goods are moved from distribution centers to fulfillment facilities and retail stores. In each vehicle, the company deployed a single ruggedized computing unit capable of processing the several terabytes of data generated daily. Using an NVMe-equipped (Non-Volatile Memory Express) edge AI inference computer, storage capacity is maximized even along with the ability to process a range of edge AI workloads.
With terabytes of autonomous vehicle data being generated, the system simultaneously analyzes data for AI inferencing and stores the data in real-time. The system has been in operation for over a year with no failures to date and is now being deployed in other autonomous projects.
The trucking company’s AI-enabled vision system integrates several advanced technologies to ensure safe and efficient autonomous deliveries. The system employs 14 cameras, which capture both color (RGB) and depth (D) information in high definition. This combination allows the vehicle to perceive and interpret its surroundings in a detailed manner, identifying objects and their spatial relationships accurately.
In addition to cameras, the machine vision system also uses six LiDAR (Light Detection and Ranging) and six radar (radio detection and ranging) sensors. LiDAR emits laser beams to measure distances by analyzing the time it takes for the light to return after hitting an object. Radar measures the distance or range of the object by using electromagnetic waves. These key sensor technologies create precise, three-dimensional maps of the environment, enabling the vehicle to detect obstacles and navigate complex routes with high accuracy, or more specifically, level 4 autonomy classification for autonomous vehicles.
The data collected from these sensors is processed in real-time by the vehicle's onboard computing system. Advanced algorithms analyze the sensor inputs to create a dynamic model of the environment, which is used for path planning and decision-making. The system can identify and respond to changes in the environment, such as moving vehicles and pedestrians, by updating the vehicle's path and speed accordingly. This data flow ensures that self-driving delivery trucks can operate safely and efficiently on fixed, repeatable routes, even in dynamic and complex scenarios.
Related: IoT Embedded Vision—What Is It and Where Can it Be Used?
Semiconductors, Heterogenous Architectures, and Edge AI
Semiconductors are foundational to enhancing AI capabilities at the edge, enabling efficient real-time processing and power management. Modern semiconductor designs often feature heterogeneous computing architectures, integrating advanced algorithmic capabilities essential for advancing edge AI.
Heterogeneous computing architectures—utilizing different types of processors and accelerators within a single system—optimize edge AI performance for specific tasks. This optimization results in more efficient processing, lower power consumption, and improved handling of AI workloads, making it ideal for edge computing scenarios where power efficiency and performance are critical.
Streamlining Fan Entry at a Sports Complex
In this system, biometric facial recognition technology leverages imaging, AI and advanced algorithms to identify individuals by analyzing their facial features. One major advantage it offers is enhanced onsite security. Traditional ticketing methods can be prone to fraud, with counterfeit tickets and unauthorized access being common issues. Facial recognition, however, ties a ticket directly to an individual’s unique facial features, significantly reducing the risk of ticket fraud and ensuring that only legitimate ticket holders can gain entry.
Another benefit is the improvement in user experience. Facial recognition allows for faster and more efficient entry into venues. Instead of waiting in long lines to have tickets manually checked, attendees can simply have their faces scanned, which speeds up the entry process. This convenience is especially valuable in large venues, such as stadiums, where quick and seamless entry is essential to managing large crowds effectively.
Related: An Overview of Ruggedization for Machine Vision Lenses
Increasing Impact on the Edge: 5G, IT/OT Convergence, and Integrated Security
In addition to heterogenous computing architectures, AI and vision technologies, other advancements are creating an impact on the rugged edge as well. For example, 5G technology significantly enhances edge computing by providing low latency and high bandwidth, up to 100 times faster than 4G LTE networks. This improvement boosts edge device performance, increases data processing capabilities, facilitates scalability, and enhances data protection. 5G enables more efficient data processing at the edge, supporting advanced applications and real-time responsiveness.
IT/OT convergence is another critical factor driving the adoption of industrial edge computing. This phenomenon merges information technology (IT), which focuses on computing, networking, and data management, with operational technology (OT), which handles real-time monitoring and control systems. Productivity and operational efficiency are enhanced based on the availability of comprehensive insights and access to proactive and predictive operational strategies. Mission-critical reliability and 24/7 functionality are required, pointing developers to rugged edge system design.
Security requirements often place great challenges on the design options used in these types of mission-critical settings. Edge computing processes data locally, reducing the need to transmit sensitive information over long distances and decreasing exposure to potential cyber threats. Edge systems are well-integrated with advanced security technologies and hardware-based measures like Trusted Platform Module (TPM) and secure boot protocols to further protect against unauthorized access and data breaches.
Related: GPU Inference Analysis: Handling Automotive Big Data for AI-Powered Machine Vision
Driving the Growth of the Edge Computing Market
The rapid adoption of edge computing is driven by a range of factors, starting with increasing demand for low-latency processing and real-time decision-making. Advancements in AI and IoT technologies are proliferating in sync, proving attractive to all types of industrial sectors and the embedded OEMs and ODMs that serve them. There is a recognized, industrywide quest for scalability and cost efficiency through better resource allocation. At the same time, hardware-based solutions are expected to enhance security and address data privacy needs— furthering their value in innovative new edge applications. The ongoing rise of Industry 4.0 technologies necessitates quick and reliable data processing, creating a competitive environment hungry for smart automation applications.
All these factors are driving an edge computing future, poised for ongoing and significant advancements. As 5G networks proliferate, industrial users will have access to reduced latency and increased bandwidth. Greater adoption of cloud-edge hybrid models will power more flexible and scalable solutions. And continued emphasis on AI-driven applications will steadily lead to smarter and more autonomous systems.
Related: EdgeX Foundry Delivers Framework for IoT Edge Computing
We’re in a transformative time with edge computing garnering global interest, particularly when integrated with AI technologies. The synergy between AI and edge computing is expected to deepen, leading to more intelligent, secure, and efficient solutions that push beyond the limits of current technology. Designers and their rugged edge partners must be equipped for a future at the edge, collaborating and building a stronger understanding of the critical considerations for durable and reliable performance in any environment
About the Author
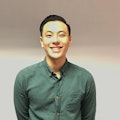
Dustin Seetoo
Dustin Seetoo is the director of product marketing at Premio (City of Industry, CA, USA). Seetoo crafts technical product marketing initiatives for industries focused on the hardware engineering, manufacturing, and deployment of industrial internet of things (IIoT) devices, and x86 embedded and edge computing solutions.