June 2019 snapshots: Autonomous vehicles, iPhone-based eye screening tool, NASA robots, infrared imaging
Cornell University study suggests stereo camera systems could replace LiDAR
Researchers at Cornell University (Ithaca, New York, USA; www.cornell.edu) have presented evidence that inexpensive stereo camera setups could provide nearly the same accuracy as the expensive LiDAR systems that are currently used in the most common approaches to developing autonomous driving technology.
In a new research study (http://bit.ly/VSD-COR) titled “Pseudo-LiDAR from Visual Depth Estimation: Bridging the Gap in 3D Object Detection for Autonomous Driving,” the authors describe a new technique by which to interpret data gathered from image-based vision systems. This new form of data interpretation, when fed into algorithms that normally are used to process information gathered from LiDAR systems, greatly increases the accuracy of image-based object detection.
The need for deploying multiple sensor systems in autonomous vehicles is understood, as demonstrated by recent initiatives like the KAMAZ (Naberezhnye Chelny, Russia; https://kamaz.ru/en/) autonomous truck project and TuSimple’s (Beijing, China; www.tusimple.com) pairing of LiDAR, radar, and camera systems in its autonomous truck system. Addressing LiDAR’s difficulty with fog and dust is also the subject of recent research conducted at MIT (Cambridge, MA, USA; www.mit.edu). The Cornell study suggests that, at the very least, stereo camera systems could provide inexpensive backup systems for LiDAR-based detection methods.
The quality of the point clouds generated by LiDAR and stereo camera depth estimators are not dissimilar, argue the researchers. However, algorithms that use image-only data achieve only 10% 3D average precision (AP), whereas LiDAR systems achieve 66% AP, as measured by the KITTI Vision Benchmark Suite developed by the Karlsruhe Institute of Technology (Karlsruhe, Germany; www.kit.edu) and Toyota Technological Institute at Chicago (Chicago, IL, USA; www.ttic.edu).
The researchers suggest representation of this image-based 3D information, and not the quality of the point clouds, is responsible for LiDAR’s comparatively-superior performance. LiDAR signals are interpreted into a top-down, “bird’s-eye view” perspective, whereas image-based data is interpreted in a pixel-based, forward-facing approach that distorts object size at distance and thus makes 3D representation more difficult the further away the data was gathered from the cameras.
The solution hit upon by the Cornell researchers was to convert the image-based data into a 3D point cloud like that produced by LiDAR, and to convert the data into a bird’s-eye view format, prior to feeding the data into a 3D object detection algorithm normally used to interpret LiDAR data. The experiment used 0.4 MPixel cameras. The result still did not equal the 66% AP achieved by LiDAR. The AP of the image-based data was improved to 37.9%, however. The researchers suggest that higher-resolution cameras may improve the results even further.
Wholesale replacement of LiDAR by stereo camera systems is not yet achievable but, according to the research published by Cornell, is possible. The researchers further suggest that if LiDAR and image-based systems were both present on the same vehicle, that the LiDAR data could be used on a consistent, ongoing basis to train a neural network designed to interpret image-only-based 3D data, thus improving the accuracy of vision-based systems as backup for primary LiDAR systems.
iPhone-based screening tool used to prevent vision disorders in children
An iPhone app called GoCheck Kids created by Gobiquity, Inc. (Scottsdale, AZ, USA; www.gocheckkids.com) eliminates the need for pediatricians to purchase specialized equipment to conduct photoscreening tests on children, a popular method for preventing the development of serious vision disorders.
Photoscreening is a vision screening technique performed on children that uses a camera and flash to detect risk factors for amblyopia, a visual impairment that causes reduced vision in one eye. The technique works by analyzing the configuration of the crescents of light returning after a camera flash and estimating refractive error, or a problem with light focusing on the back of the eye. The images can be analyzed manually, or by software. The technique has become popular in recent years as an option to replace screening with traditional eye charts, especially for children who refuse to cooperate with an eye chart screening. Most photoscreening is conducted with specialized devices.
The GoCheck Kids app instead uses an iPhone as the delivery method for the photoscreening. When a child goes in to the pediatrician for a checkup, the doctor takes an iPhone picture of the child from a distance of 3.5 feet, and machine learning software in the app reviews the captured image to detect warning signs foramblyopia.The GoCheck Kids app is made possible by Core ML software developed by Apple (Cupertino, CA, USA; www.apple.com), which enables integration of machine learning models into iPhone apps, and includes software called Vision, which can apply computer vision algorithms to image-based iPhone tasks. Vision’s framework performs face and face landmark detection, among other routines, and allows the creation of models for classification and object detection.
The efficacy of GoCheck Kids was measured in a study (http://bit.ly/VSD-VAPP) titled “Evaluation of a smartphone photoscreening app to detect refractive amblyopia risk factors in children aged 1-6 years.” The results analyzed were drawn from data generated by an iPhone 7 in portrait mode. Pictures were taken of 287 children. The images were reviewed by the GoCheck Kids image processing algorithm, and manually by ophthalmic image specialists using calipers. The inspection results were graded by the gold standard 2013 American Association for Pediatric Ophthalmology & Strabismus (San Francisco, CA, USA; www.aapos.org) amblyopia risk factor guidelines.
The overall sensitivity and specificity for detecting risk factors by the app was 76% and 85% respectively via manual grading, and 65% and 83% respectively for the app-graded results. The study concluded that GoCheck Kids is a viable photoscreening delivery method for detecting amblyopia risk factors. More than 4,000 pediatricians have conducted a total of 900,000 screenings with GoCheck Kids and identified risk factors in over 50,000 children.
NASA’s new robots for the ISS feature multiple cameras
New robotic assistants called “Astrobees,” developed by NASA (Washington, D.C., USA; www.nasa.gov) to assist in the operation of the International Space Station (ISS), are equipped with a sizeable payload of cameras and sensors to allow them to navigate the station.
The three Astrobees deployed to the ISS, named Honey, Queen, and Bumble, are cube-shaped robots that measure 32 cm wide. They were developed at NASA’s Ames Research Center in Moffett Field, CA, USA to help astronauts with maintenance, inventory tracking, conducting experiments, and studying how humans and robots will interact in space.
The Astrobees are propelled by a pair of battery-operated fans that allow movement in any direction in zero gravity. They are equipped with a robotic arm for gripping objects and handrails. When an Astrobee’s battery is low, it will automatically fly to its power station and dock to recharge. The robots can function autonomously or via remotecontrol.The Astrobee uses six commercial, off-the-shelf external sensors for navigation. A fixed-focus color camera with wide field of view called the NavCam streams footage to the robot’s control station at 1 Hz. An auto-focus color camera called the SciCam that downlinks live HD video, and a LiDAR anti-collision system called the HazCam, are also located on the forward face of the Astrobee.
On the aft (rear) side of the Astrobee is the DockCam, a color camera almost identical to the NavCam, that provides guidance for the Astrobee during docking maneuvers with its recharge station. The DockCam reads fiducial markers, or objects used as points of reference, to help guide the Astrobee into its dock. The Astrobee can connect to its dock with a hard-wired Ethernet connection to download logs of the robot’s activity, as backup for operational data streamed to Earth control stations while the Astrobee is on duty.
The PerchCam, which is identical to the NavCam, is also located aft and turns on to detect handrails on the ISS. The Astrobee perches on handrails to reduce power consumption and get out of astronauts’ way during operations. Finally, the Astrobee has a top-facing sensor package called the SpeedCam that uses infrared imaging, an inertial measurement unit, and traditional optics to estimate velocity and control speed in order to avoid collisions.
The Astrobee uses its vision system to navigate the US Orbital Segment (USOS), the components of the ISS built and operated by the United States, by comparing images of the ISS interior with an onboard map of the station. Because the robot’s cameras are always on, the ISS crew is made aware of when the Astrobee is streaming audio or video via LEDs on the fore and aft that indicate whether the robot is streaming video and audio, or only audio.
The NASA Astrobee Research Facility will make the Astrobees available to guest scientists for zero-gravity robotics research on the ISS.
Spider monkey population monitored by drones with thermal infrared cameras
Researchers outside of Tulum, Quintana Roo, Mexico deployed drones equipped with thermal infrared (TIR) cameras to monitor spider monkey populations in the Los Arboles Tulum residential development.
Spider monkeys are categorized as Endangered or Critically Endangered on the IUCN (Gland, Switzerland; www.iucn.org) Red List of Threatened Species. They live in the forest canopy, tend to split into subgroups with shifting size and distance among members, and can move among the trees very quickly. These challenges make spider monkeys difficult to count via ground observation and successful conservation efforts depend on frequent, accurate population counts.
In their study (http://bit.ly/VSD-SPID), titled “Thermal Infrared Imaging from Drones Offers a Major Advance for Spider Monkey Surveys,” published on March 11, 2019, researchers compared ground surveys of spider monkey population with counts from drones equipped with TIR cameras. The researchers used a TeAx Technology (Wilnsdorf, Germany; www.teax.de) Fusion Zoom dual vision camera containing a FLIR (Wilsonville, OR, USA; www.flir.com) Tau2 640 core infrared camera with 19 mm lens, and a 1080p Tamron (Saitama, Japan; www.tamron.com) RGB camera, mounted side-by-side on a drone via a 2-axis stabilization gimbal to provide camera steadiness duringflights.The drone was a 550 mm quadcopter frame built from extruded aluminum arms and glass fiber plates, with a 10-minute battery-powered flight time. Open-source ArduCopter (www.arducopter.co.uk) firmware ran a Pixhawk 2.1 autopilot configured for manual or automatic flight. Grid pattern searches were programmed with the Mission Planner application for ArduPilot (www.ardupilot.org).
The Tau2 camera was used as a handheld device for preliminary surveys to determine how the monkeys would appear in the drone footage and to gauge their surface temperature, which was determined to be 30° C. The researchers also generated a typical, 24-hour temperature range for the forest based on several years’ worth of satellite observations. They determined that drone flights had to be conducted between around sunset and before sunrise in order to provide the needed thermal contrast between monkey and environment.
Twenty-nine grid pattern searches were conducted over the monkeys’ three most-used sleeping sites. Four flights were also conducted in which the drone hovered for a minimum of five minutes over one of the monkeys’ sleeping trees. TIR footage was analyzed by an expert in observing warm-blooded animals via infrared imaging. Five experienced observers equipped with binoculars and GPS units conducted ground counts.
The study found that for subgroups of fewer than 10 spider monkeys, ground and drone counts were generally in agreement. Where counts of subgroups of more than 10 spider monkeys were concerned, however, drone counts were higher than ground counts in 92% of cases. In addition, 75% of cases in which ground observers missed monkeys in large subgroups occurred at one of the sleeping sites where view from the ground is more obscured by vegetation compared to the other sites, an issue that did not affect the drones and TIR cameras. The mean increase in number of monkeys detected by drone flights versus ground observation was 49%.
The researchers pointed out that heterogenous vegetation, mistaking mothers and young as single heat signatures, and regulations against beyond visual line of sight flights could make large-scale surveys a challenge.
About the Author
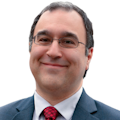
Dennis Scimeca
Dennis Scimeca is a veteran technology journalist with expertise in interactive entertainment and virtual reality. At Vision Systems Design, Dennis covered machine vision and image processing with an eye toward leading-edge technologies and practical applications for making a better world. Currently, he is the senior editor for technology at IndustryWeek, a partner publication to Vision Systems Design.