Machine learning system and IR cameras mounted on drones successfully identify koala populations
A group of scientists from the Queensland University of Technology in Australia have developed a system to detect koala populations using algorithmic analysis of infrared footage captured by drone flights.
Koalas are listed as vulnerable, not endangered, in Australia. However, the eucalyptus forests that are the koalas' primary habitat are threatened by the spread of agriculture and urban construction. Monitoring koala populations is therefore important for conservationists and the task is difficult because koalas inhabit a wide area and live in environments covered by forest canopies.
In their paper, "Automated detection of koalas using low-level aerial surveillance and machine learning," published on March 1, 2019, the scientists on the project cite that typically only 60%-75% of koalas present within a survey area are detected through ground observation and photo imaging.
Enter the drones, infrared cameras, and machine learning. For their study, the scientists used FLIR Tau 2 640 thermal cameras with a resolution of 640 x 512, 13 mm focus length lens, and 9 Hz frame rate, mounted onto a DJI Matrice 600 Pro drone equipped with the A3 Pro flight controller. Thermoviewer was used to process the drone footage and the Faster-RCNN and YOLO object detection deep convolutional neural networks (DNNs) were used to detect the koalas.
"There are a number of factors around the drones/sensor, but as a university with a number of different applications the FLIR seemed most versatile, and the 600 Pro had good flight times and capacity to carry reasonable loads," said Dr. Grant Hamilton, one of the authors of the study.
Each DNN was fed previously-captured thermal data from drone flights where the koala locations had been confirmed via radio tracking and manual inspection. This data allowed the DNNs to generate models by which to identify images in analyzed footage as potential koalas. The models were applied to test footage and the results were manually corrected via identifying the locations of koalas present in the footage but not detected by the models and marking false detections as negative results.
Eucalypt forests in north and south Petrie Mill, Queensland were chosen as the experiment sites, with a known population of 48 koalas that were surveyed by a ground team on the same day as the drone flights would take place, in order to validate identifications made by the DNNs. Eleven drone flights were conducted between February and August 2018.
The DNNs separately drew heat maps of potential koala locations and the data was compared to determine consistent results between the two networks. The ORB (Oriented FAST and Rotated Brief) algorithm developed by OpenCV labs was used to assist in this process by accounting for change in images resulting from movement of the drone. If potential koala heat signatures were consistent over enough concurrent frames the signatures were accepted as detections. The data was then manually reviewed.
The automated, DNN-driven detection method had an 87% overall probability of successfully identifying a koala from the infrared footage, whereas manual inspection of the thermal data resulted in a 63% probability. The automated system required an average of 136 minutes to process the thermal imaging and identify potential koalas. An average of 170 minutes was required to manually sift through the data and identify probable koala locations.
The scientists posit that if the DNNs had been trained appropriately that the procedure could be used to identify multiple types of animals from a single batch of thermal footage, and that use of the method to detect other types of animals would help validate the results of the koala test.
Related stories:
FLIR Systems provides thermal cameras for anti-poaching efforts in Kenya
3D vision system monitors the behavior of pigs
Autonomous underwater vehicle designed to destroy harmful starfish successfully deployed
Share your vision-related news by contacting Dennis Scimeca,Associate Editor, Vision Systems Design
To receive news like this in your inbox, click here.
About the Author
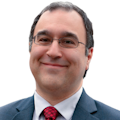
Dennis Scimeca
Dennis Scimeca is a veteran technology journalist with expertise in interactive entertainment and virtual reality. At Vision Systems Design, Dennis covered machine vision and image processing with an eye toward leading-edge technologies and practical applications for making a better world. Currently, he is the senior editor for technology at IndustryWeek, a partner publication to Vision Systems Design.