Face recognition algorithms fail in the post-COVID-19 world
According to a new study published by the National Institute of Standards and Technology (NIST) (Gaithersburg, MD, USA; www.nist.gov/), part of the U.S. Department of Commerce (Washington, DC, USA; www.commerce.gov/), face recognition algorithms produced prior to the COVID-19 pandemic can suffer up to 50% authentication failure rates when the subjects wear face masks.
Wearing masks in conjunction with social distancing proves effective in preventing the spread of the novel coronavirus. Therefore, a face recognition system currently may need to conduct operations with primary facial features like noses and mouths obscured from view.
The NIST study (bit.ly/VSD-NISTMSK) ran under the Ongoing Face Recognition Vendor Test (FRVT) standard established by the agency, against a set of photos used for FRVT benchmarks since 2018, drawn from unmasked application photographs of persons applying for immigration benefits and border crossing photographs of travelers entering the United States.
Applying digital masks to the border crossing photos allowed controlled exploration of varying factors like mask shape, color, and amount of nose coverage. The technique also allowed production of very large datasets. The application photographs did not receive masks, to mimic a scenario where a person wearing a mask attempts to authenticate against a prior visa or passport photo. More than 6 million images of over 1 million people tested against 89 face recognition algorithms.
Related: Deep learning powers chimpanzee face recognition research
The study determined that the most accurate algorithms suffered 5% authentication failure rates against images when 70% of the face occluded by a mask. Other algorithms failed to authenticate between 20% and 50% of images. False negatives can normally be mitigated by second attempts that correct pose, expression, or illumination. A false negative created by a subject wearing a mask, however, will not be resolved by a second attempt because the failure condition cannot be remedied.
Mask shape affected performance. Full-face-width masks covering more of the face than a rounder N95-type mask, resulted in a false negative rate twice that of rounder-type masks. The study considered light blue and black mask colors, with black masks providing higher error rates. Some algorithms couldn’t detect a face entirely when the subject wore a mask.
NIST stresses that the study does not account for possible algorithm fine tuning, or the effect of human examination’s ability to correct false positives. Nonetheless, while NIST only measures algorithm performance and does not make recommendations for future action, its data suggests that new post-pandemic algorithms require development to allow face recognition systems to provide accurate results in the pandemic environment.
Image credit: B. Hayes/NIST
About the Author
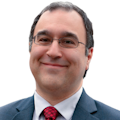
Dennis Scimeca
Dennis Scimeca is a veteran technology journalist with expertise in interactive entertainment and virtual reality. At Vision Systems Design, Dennis covered machine vision and image processing with an eye toward leading-edge technologies and practical applications for making a better world. Currently, he is the senior editor for technology at IndustryWeek, a partner publication to Vision Systems Design.