Monitoring Critical Infrastructure with AI-Based Computer Vision
While computer vision is already well established in manufacturing and surveillance applications, it can also be implemented to monitor critical infrastructure such as roads, bridges, railway tracks, pipelines, power lines, oil and gas tanks, and dams. The latest developments in machine learning, embedded computing, and drones open new possibilities for the comprehensive inspection of such equipment for predictive maintenance, even in remote locations.
Ensuring Safety and Reliability of Critical Infrastructure with Predictive Maintenance
On August 14, 2018, the Ponte Morandi, a major highway viaduct in Genoa, Italy, collapsed during a rainstorm, killing 43 people. It was not only a disaster for the lost lives and injured people, but also had dramatic consequences for the region’s economy as Genoa is one of Italy’s largest harbors.
This example shows how critical infrastructure is vital to our society, supplying people with energy, water, information, and ensuring the mobility of people and goods. Failure can have dramatic consequences, whether in the form of lethal accidents, power outages, floods and destruction, or the interruption of healthcare services. It can also cause severe damage to the environment, for example, through pollution or fire if an oil pipeline leaks.
In Genoa, insufficient maintenance was suspected to be a cause for the disaster. Continuous monitoring to predict issues and prevent damage is essential for such infrastructure. However, performing this type of inspection is a particularly challenging task.
Whether a bridge, a rail track, a power line, or a pipeline, most critical infrastructure is substantial, such as a very large building or piece of equipment that stretches over hundreds or thousands of miles. For example, the Canadian Nova Gas Transmission Ltd. (Calgary, AB, Canada) operates a natural gas gathering and transportation system including approximately 24,500 km of pipelines (more than 15,000 miles) in Alberta and northern British Columbia, according to the Canada Energy Regulator (Calgary, AB, Canada). Sending staff on-site to perform a seamless inspection is a very difficult, time-consuming, and costly endeavor. Deploying cameras and vision systems over such a distance is also a huge investment.
Related: Remote Bridge Health Monitoring Provides Insights into Structures
Additionally, infrastructure is sometimes located in hostile environments, for example offshore, in remote northern territories of Canada or in the Arabian desert, far from human settlements, a power grid, or telecommunication networks. This makes it more difficult to install automated inspection systems based on cameras and PCs.
Computer Vision for Predictive Maintenance
Because of these specific constraints, there is no way predictive maintenance of such infrastructure can be performed manually. Computer vision can automatically detect and report defects or issues using various technologies. Here are some examples:
● Surface inspection can detect material damage, cracks, corrosion, and other defects.
● Infrared and multispectral imaging can help identify internal material fatigue that is not visible at the surface or detect leaks through the temperature difference between the gas or liquids conveyed by a pipeline and the outside.
● Thermal imaging can provide information on the overheating of electrical equipment, for example, as a result of insulation issues. It can also monitor the temperature of chemical reactions from outside a tank in a chemical plant or oil refinery.
● Environmental and aerial imaging can identify potential threats to the infrastructure from the natural surroundings—e.g. a landslide or vegetation damaging power or telecommunication lines.
Related: Low Power Image Sensors for Modern Vision Systems
The hardware and software vision technology necessary to perform these tasks is already well established. The challenge lies in the scale and the conditions in which such monitoring must take place. PC-based machine vision systems require a lot of space, power, and maintenance and are not easy to deploy in hostile outdoor environments with extreme temperatures and weather conditions. Furthermore, their cost is prohibitive when deployed on a large scale.
Embedded Systems and AI Image Processing Lower the Barrier of Entry
The recent developments in embedded systems and AI-enabled image processing open new opportunities for operators and regulators of such infrastructure. Embedded processors, such as from Arm (San Jose, CA, USA) or NVIDIA (Santa Clara, CA, USA) now offer enough processing capacity to perform advanced image processing tasks within a small footprint, with less power, and at a lower cost than PC-based systems. The favorable SWaP-characteristics (Size, Weight, and Power) of embedded vision systems make it easier to package them in compact protective housings to withstand outdoor conditions. They can easily be mounted on vehicles such as maintenance trains scanning the tracks or trucks monitoring road condition. They can also be mounted on drones and airplanes for aerial imaging of long-range infrastructure.
Related: When to Use Edge Learning in Machine Vision Applications
For pipelines, for example, monitoring is performed either via aerial imaging or from inspection towers located at a regular distance along the course of the pipeline. Outdoor cameras mounted on top of a tower or mast are not easy to access for power supply, maintenance, or to read out data. Low-power embedded systems can be battery powered with the battery itself being loaded by a small solar panel. Since the complete image processing happens within the camera, no data cables or wireless connection to a remote CPU are needed for the image processing itself. The system can communicate the outcome of the image analysis as low bandwidth data via mobile networks or satellite communication—for example, transmitting the information of a leak and its GPS coordinates rather than Gigabytes of image data.
Autonomous Ice Prevention with Embedded AI Vision System
In cold regions, ice formations can damage power or telecommunication cables or other infrastructure such as buildings. Ice dams on building roofs can cause severe damage to the infrastructure by preventing melted water from draining properly. Electrical heat cables placed at critical locations can melt ice blocks before they cause damage. However, operating them 24/7 is a massive waste of energy and generates unnecessary costs.
A U.S.-based heat cable provider offers camera-monitored heat cables that rely on a fully autonomous embedded vision system. The smart camera module fits in a 76 x 178 mm (3 in x 7 in) ruggedized housing that protects it from weather conditions. It includes a battery and miniature solar panel, so it does not need to be connected to the power grid. The device has been optimized for low power consumption, so a fully loaded battery is enough for weeks of operation, even in low-light conditions—for example, if the camera and the solar panel are covered with snow.
The system continuously monitors the target area and triggers heat cables automatically when it detects ice formations beyond a given threshold. As soon as the ice has melted, the heat cables can be safely switched off.
This application is a perfect example of how an AI-based embedded vision system can be deployed at low cost and with little effort and operate fully autonomously. It not only detects issues and triggers an alarm for human intervention, but even goes further by initiating corrective actions automatically. On top of that, it optimizes the cost of operation by minimizing energy consumption and saving tons of CO2 emissions through wasted power.
Large-Scale Predictive Maintenance Requires Robust, Low Cost and Low-Power Solutions
Critical infrastructure brings its own challenges when it comes to predictive maintenance: outdoor imaging, remote locations, limited access to power and networks, and the large scale of the objects to be inspected. This calls for either mobile solutions or a myriad of small, low-cost imaging systems for seamless coverage.
Related: How Drexel University Researchers Improved the Inspection of Concrete Structures
AI-based embedded systems are small, lightweight, affordable, and low-power. They can be embarked on inspection vehicles or mass-deployed over large distances to monitor autonomously and even trigger corrective actions automatically.
About the Author
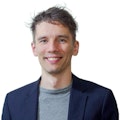
Constantine Malynin
Constantine Malynin, is co-founder of Maxlab (Edmonton, Alberta, Canada), a company that develops edge AI cameras.