Machine vision system deploys novel deep learning software
When traditional, rules-based machine vision solutions proved cumbersome for inspection applications at a BMW Group (Munich, Germany: www.bmwgroup.com) engine manufacturing plant in Steyr, Austria, the company decided to investigate the integration of a vision-based inspection system featuring deep learning software comprised of three generalist artificial intelligence (AI) models.
David Bricher, Technical Lead at BMW Group plant Steyr’s innovation and digitalization team, the InnoLab, spearheads new technology initiatives to increase the quality and efficiency of the company’s production processes. Bricher’s team currently focuses on the development of inspection solutions that can deploy in a short amount of time and only require images of good parts that pass inspection.
Bricher’s team decided to initiate a pilot program for the INSPEKTO (Raman-Gan, Israel; www.inspekto.com) S70 (Figure 1), a turnkey inspection system designed for ease and flexibility of deployment, reportedly requiring no particular machine vision expertise. Customers may remove the system from the box, image a small number of good products, and immediately set the system to work on the production line, according to Inspekto.
Related: Deep learning system powers traffic enforcement system
The package includes all the components necessary to deploy a vision system: camera, lens, lighting, platform (in this case, a PC), and software (Figure 2). Inspekto also includes an industrial controller, a mounting adaptor, and cables. The system also features Power-over-Ethernet. The components are modified versions of off-the-shelf products from major manufacturers, says Ofer Nir, VP of Products and Marketing at Inspekto.
The included greyscale, global shutter, 5 MPixel, GigE interface camera features 12X optical zoom and can trigger automatically, manually, or via PLC. The camera captures 2D images and also functions as a 2D code or 1D barcode reader, has an installation distance of 10 to 5000 cm, can inspect a part in approximately 0.5 seconds, and has a minimum defect size of 0.3 mm at 10 cm distance.
A flat dome light with 5000K LEDs controlled by pulse-width modulation, arranged in eight regional strips to provide variable lighting directionality and intensity, mounts at the front of the camera. The LEDs provide illumination on the same optical axis of the camera, to avoid creating shadows, and can provide pulsed illumination synchronized with camera exposure. Additional lights can supplement the default lighting embedded in the camera, as needed.
“In past use cases, the addition of colored lighting helped reveal color defects and scratches on PCB boards, and in other cases when the INSPEKTO S70 mounts far away from the device under inspection, requiring additional illumination,” says Yonatan Hyatt, CTO at Inspekto.
Linux Ubuntu distribution, running on the included 240 x 225 x 10.5 mm controller, provides the platform for image processing software. The controller features Ethernet and Wi-Fi network interfaces and ProfiNet, EtherNet/IP, Modbus/TCP, and digital I/O industrial connectivity options.
The BMW Group invited Inspekto to its Steyr plant for several days of proof-of-concept tests. Bricher’s team identified a viable test application for the INSPEKTO S70’s capabilities, checking the correct locking of a knock sensor for three-cylinder or four-cylinder engines that monitors the engine for sounds during combustion and determines whether ignition timing requires adjustment.
Engine blocks have many parts such as pipes and cables that can act as visual disturbances for traditional machine vision systems. The knock sensor is hardly visible, says Bricher, which adds additional difficulty for inspection via rules-based applications.
The identification of not entirely locked sensors is a critical task that is hardly noticeable with the bare human eye. In this use case the protruding of a metal strap of the knock sensor indicates an incorrect installation. The clear differentiation between good and bad images made this inspection an appropriate test.
Inspekto deploys in its products a system of generic AI models developed with unique architectures and training routines. The first AI model automatically adjusts illumination, shutter speed, and exposure to determine optimal settings to capture the highest quality image possible. During operation, the model also adjusts these settings dynamically based on changes in environmental conditions.
The second AI model detects the part to inspect, determined in the setup phase by drawing an outline around the image of a part that would pass inspection, displayed on the 21.5 in., 1920 x 1080, 60 Hz monitor included with the system. The operator can adjust the outline on subsequent images, if necessary. For the most part, says Nir, setup only requires one outlined image (Figure 3).
As setup continues, the production line runs and the system captures images of parts that the operator identifies as passing or failing inspection, using the system’s HMI. The second AI model determines how many images it needs to complete training, in 95% of cases between 20 to 30 images, says Nir. Environmental conditions such as flickering lights or vibration on the line may necessitate capturing up to 35 training images, adds Nir, but these situations are outliers in the company’s experience.
Related: Deep learning tools inspect food and organic products
Commonly in deep learning applications, an AI model trains to detect specific objects within an image through processing a multitude of training images in which the specific object is identified and labeled. Once the model knows what the object to detect looks like, the model can detect that object in fresh images.
The AI detection model developed by Inspekto, on the other hand, trained on images of hundreds of different parts, with thousands of training images for each part. The end result, a training dataset with millions of images, produces a base detection model trained not to look for any specific parts in an image but how to look for any part in an image, period. The operator then adapts the model by providing the requisite 20 to 30 training images.
Once the second AI model can detect the specific part in an image, the third AI model may conduct the actual inspection of the part by comparing the part under inspection to the training images of good parts. The third model classifies each image with a result of good or bad. The Inspekto system can only provide binary classification, not an explanation as to why a part may classify as bad, the trade-off for using an adapted, generalist model. The client must manually inspect the image and determine the nature of the anomaly.
The system has a +/- position tolerance of a few degrees on all rotation axes, and tolerance for spatial shift. Both are adaptive to the 3D structure of the object being inspected. The more complex the object, the smaller the amount of position tolerance. A part outside these tolerances generates an alert to inform the operator that the part’s location requires correction or approval. If the operator reviews good results and determines a high false positive rate, they may have to adjust the training images and repeat that aspect of the setup process.
In the case of the BMW Group knock sensor application, the INSPEKTO S70 had no trouble identifying images where the knock sensor’s metal strap only slightly protruded from the sensor and thus indicated an installation that would not pass inspection.
Based on the positive results of the pilot program, Bricher plans to educate the maintenance staff to be able in the future to install the system on their own. A final integration into the production line is scheduled for Q1 2021.
About the Author
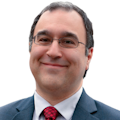
Dennis Scimeca
Dennis Scimeca is a veteran technology journalist with expertise in interactive entertainment and virtual reality. At Vision Systems Design, Dennis covered machine vision and image processing with an eye toward leading-edge technologies and practical applications for making a better world. Currently, he is the senior editor for technology at IndustryWeek, a partner publication to Vision Systems Design.