Miniature thermal camera system provides hand tracking
Accurate hand tracking represents a significant challenge in the development of accessible augmented reality and virtual reality (AR/VR) technology, human-robot interaction, and wearable user interfaces. Current solutions like cameras placed in multiple, strategic locations for tracking the hand from various angles, handheld controllers, and the sort of gloves worn for motion tracking in entertainment are inconvenient or limit mobility and therefore impractical for everyday use.
A team of researchers from Cornell University (Ithaca, New York, USA; www.cornell.edu/) and the University of Wisconsin-Madison (Madison, WI, USA; www.wisc.edu), working in the SciFi Lab at Cornell University (bit.ly/VSD-SFLAB) run by Dr. Cheng Zhang, have developed a more convenient potential option, a wristband enabling continuous 3D finger and hand pose tracking, using four miniature thermal cameras.
The research was published as a paper titled FingerTrak: Continuous 3D Hand Pose Tracking by Deep Learning Hand Silhouettes Captured by Miniature Thermal Cameras on Wrist (bit.ly/VSD-FGTRK).
The FingerTrak system (Figure 1) can inference 20 finger joint positions and comprises four 9.3 x 9.3 x 5.7 mm MLX90640 thermal cameras from Adafruit (New York, NY, USA; www.adafruit.com) capable of a 16 fps capture rate at 32 x 24 resolution, mounted 2 mm above the wearer’s skin on a wristband. FingerTrak employs a deep neural network that estimates finger joint positions in 3D space by stitching together multi-view images of the outline of the hand generated by the thermal cameras.
Image frames synchronize using a time-matching algorithm and four Raspberry Pi (www.raspberrypi.org) units transmit the captured images to a ThinkPad XI laptop from Lenovo (Morrisville, NC, USA; www.lenovo.com) with an Intel (Santa Clara, CA, USA; www.intel.com) Hexa-Core i7-8750H CPU via wireless LAN.
To help train and evaluate the system, the researchers also use a Leap Motion external depth sensor from Ultraleap (San Francisco, CA, USA; www.ultraleap.com) that captures images at 60 fps using three active infrared LEDs and two MT9V024 image sensors from ON Semiconductor (Phoenix, AZ, USA; www.onsemi.com) to generate ground truth data. The thermal images and ground truth data transmit to a GPU cloud instance with 8 K80 GPUs at Amazon Web Services (Seattle, WA, USA; aws.amazon.com).
Experimental results
A study conducted with 11 participants, 6 male and 5 female, evaluated the system. The study took place in two parts, one in which participants held nothing in their hand and another when holding an object.
In the first part of the study, the participant performed 19 different hand poses in ten different sessions. The first seven sessions were used as training sessions, and the last three were used as testing sessions. Two different backgrounds were used for the test, a whiteboard with post-it notes and a shelf with books. In each training session the researchers slightly changed the position and orientation of the wristband, to generalize the model.
In the second part of the study, the subjects held objects to deliberately prevent the camera from having a complete, constant view of all five fingers and therefore test the model’s robustness. Each subject held for four seconds a smartphone, a book, an apple, and a pen, in random order. The first 32 sessions were used for neural network training and the last 8 sessions for testing.
Leap Motion cannot provide reliable hand tracking to generate ground truth images when the hand holds an object. Therefore, every time a test subject held an object, the researchers asked the subject to maintain their hand position while removing the object from their grasp, allowing Leap Motion to generate the ground truth image.
Related: 4D tracking system simultaneously recognizes the actions of dozens of people
The researchers created from each test subject 10,000 image samples for training and 2,000 image samples for testing, by sampling key frames from the videos recorded during the test sessions. Each sample contained four thermal images captured simultaneously, corresponding to the four thermal cameras on the wristband. User-dependent, individual models were trained for each test participant
FingerTrak prediction quality was compared to reference hand poses obtained from Leap Motion, and measured in terms of mean absolute error (MAE) for joint positions and joint angles. When tracking an empty hand against the same background, the FingerTrak system demonstrated a MAE of approximately 1.2 cm for joint positions and 6.46° for joint angles, across all 11 test participants.When tracking an empty hand against the same background, the FingerTrak system demonstrated an MAE of approximately 1.2 cm for joint positions and 6.46° for joint angles, across all 11 test participants.
Background challenges
An empty hand against different backgrounds presented more challenging test conditions. MAE for joint positions and joint angles increased to 2.09 cm and 8.06° respectively. The researchers conjecture that the increased error rates owed to camera shift during large arm motion when the participant moved the wristband to the new background. Similarly, in tests where the device was taken off and re-mounted on a participant, MAE for joint positions and joint angles further increased to 2.72 cm and 9.44° respectively. Both results demonstrate the importance of stable positions for the thermal cameras.
When the participant held an object, MAE for joint positions increased to 2.68 cm and for joint angles to 10.37°. Considering the difficulty of a system like Leap Motion to provide hand tracking at all when an object is held, the researchers consider these results promising and will move on to testing with a larger set of objects.
Upgrading to higher-resolution cameras that better differentiate the thermal signatures of hand outlines from backgrounds, applying segmentation routines to separate the hand outline from background images, exploration of different deep learning architectures, and using large-scale synthetically generated training data may all provide accuracy improvements.
A better ground truth acquisition method than Leap Motion could also improve the system, according to the researchers, as the current method cannot recognize complicated hand poses, which affects the quality of model training. Using a glove changes the shape of the hand and therefore could provide inaccurate ground truth data, however.
Finally, given the testing results where the position of the cameras shifted, lighter-weight cameras that do not shift as much during arm motion, or including in training datasets images that result from camera shift, may address this issue. Furthermore, a calibration gesture or further augmentation of the training dataset may help FingerTrak account for changes when removing and re-mounting the system.
User-independent models produced much worse performance than user-dependent models. This consideration applies to the efficacy of deploying a system such as FingerTrak widely in a commercial setting, where individual users may lack the resources to generate training and testing datasets and create their own, individual models. Critical topics for future research include determining methods for creating an accurate, generalized model and developing a large enough training dataset to address the problem.
About the Author
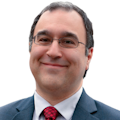
Dennis Scimeca
Dennis Scimeca is a veteran technology journalist with expertise in interactive entertainment and virtual reality. At Vision Systems Design, Dennis covered machine vision and image processing with an eye toward leading-edge technologies and practical applications for making a better world. Currently, he is the senior editor for technology at IndustryWeek, a partner publication to Vision Systems Design.